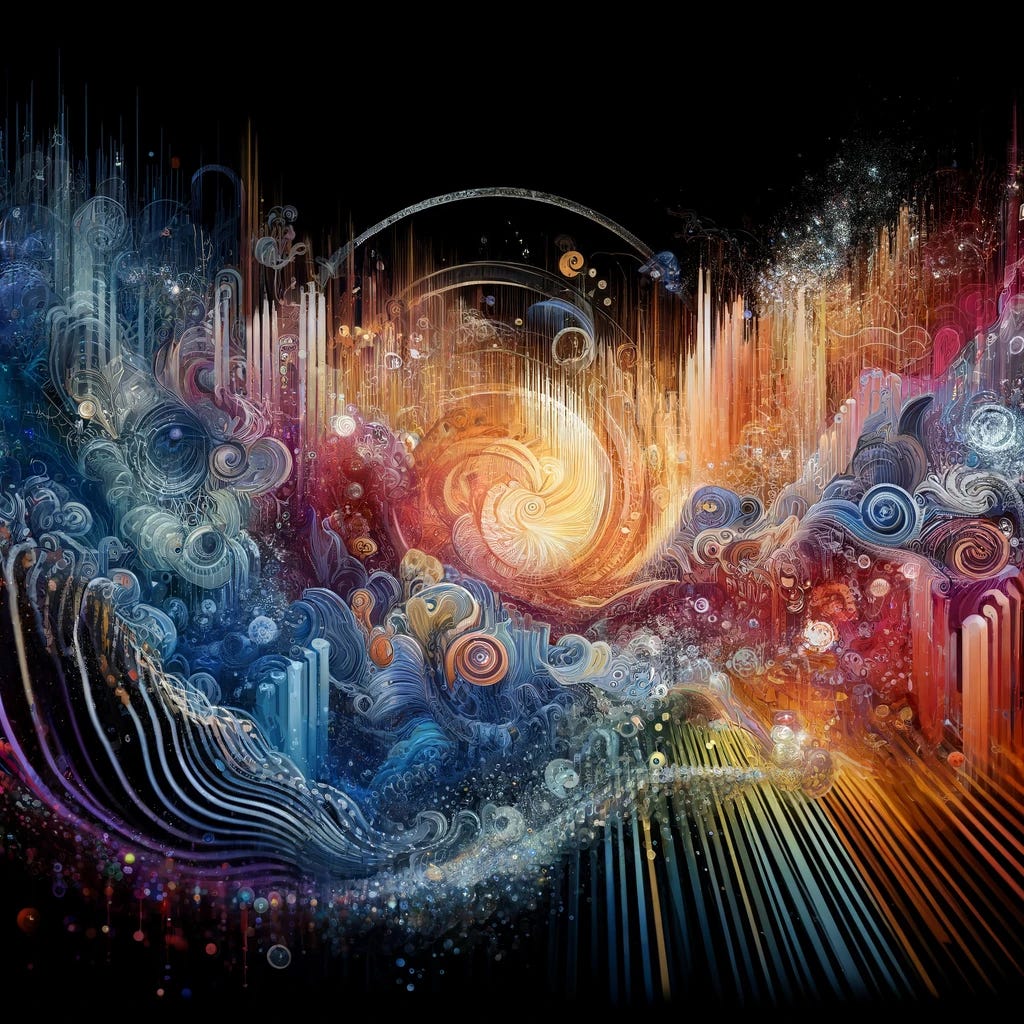
Episode 140 β April 4th, 2024 β Available at read.fluxcollective.org/p/140
Contributors to this issue: Scott Schaffter, Jon Lebensold, Kamran Hakiman, Erika Rice Scherpelz, Neel Mehta, Boris Smus, MK, Ben Mathes
Additional insights from: Ade Oshineye, Justin Quimby, Dimitri Glazkov, Alex Komoroske, Robinson Eaton, Spencer Pitman, Julka Almquist, Lisie Lillianfeld, Samuel Arbesman, Dart Lindsley, Melanie Kahl
Weβre a ragtag band of systems thinkers who have been dedicating our early mornings to finding new lenses to help you make sense of the complex world we live in. This newsletter is a collection of patterns weβve noticed in recent weeks.
βWe always hope for the easy fix: the one simple change that will erase a problem in a stroke. But few things in life work this way. Instead, success requires making a hundred small steps go right β one after the other, no slipups, no goofs, everyone pitching in.β
β Atul Gawande
ππ§ Numberwang-driven development
On a scale of 1 to 5, how was your burrito? On a scale of π to π , how was the bathroom? Whether itβs a 10-point scale for evaluating your most recent LEGO purchase or a 7 question CSAT survey evaluating the efficacy of a complex product, distilling the world down to a numeric scale can provide a sense of actionable legibility. Taken to an extreme, it can also drive us to make unmoored decisions. Over-indexing on quantitative measures can lead us to undervalue qualitative measures, make decisions that actively detract from usersβ experiences, and add noise to our signal. Letβs unpack some of these effects.
Qualitative ways of knowing can be extremely powerful. Think of when you played LEGO with kids or parents, rode a bike for the first time, or watched a sunset. Distilling these experiences down to a 5-point scale does not capture the joy and value of those experiences. If we only focused on those quantitative scores, weβd create a strange dystopian world where the only thing that matters is our rating (Γ la Black Mirror). This applies to work as well. Even when the questions were inspired by quantitative metrics, talking to our users is likely a better means of finding product insights than trying to infer them from the metrics alone. Doing so may allow simplifying our metrics strategy, focusing instead on βvibes based developmentβ β Max Rozenβs term for a process in which he asks users one question and then follows up with a more in-depth conversation.
When used indiscriminately, adding quantitative measures can detract from the product experience. Imagine if we were not allowed to exit a movie without providing a rating. This may seem farcical, but we do seem to build similar dynamics into product flows β think of how many NPS score popups youβve closed lately. Removing measurements that slow down the user experience can directly improve that experience. That doesnβt mean removing every measureβ¦ but we should be aware of how our users experience our products.Β
Finally, quantitative metrics can increase noise in the system. Goodhartβs law comes into play rapidly. Over-focusing on a single, counterbalance-free metric as a goal gets gamed β and, as a result, takes on a life of its own. Even if the metrics are good, the sheer number can make them less meaningful: trying to create meaning from 50 metrics is substantially harder than for 3. And when we have many metrics, that makes it easier to advocate for adding even more. This leads to an all-too-common situation where product owners argue for yet another metricβ¦ which they never actually use to make decisions. Measuring more doesnβt necessarily help in making better decisions.
Making data-driven decisions isnβt necessarily a bad thing. Just be sure itβs not the only thing. Qualitative expertise is needed to counterbalance metrics and to pick and design which metrics to use. Metrics are not magic; theyβre just one observational tool at our disposal. The next time you find yourself in a meeting reminiscent of the fictional game show Numberwang, take a step back and evaluate the full spectrum of approaches you can use to make decisions.
(By the way, you might enjoy our latest fascination: making suno.ai songs for our articles.)
π£οΈπ© SignpostsΒ
Clues that point to where our changing world might lead us.
ππ―π΅ Japan became the last country to end negative interest rates
Japanβs central bank recently announced its first interest rate hike in 17 years, from -0.1% (meaning companies would have to pay to keep their money in a bank) to 0.0β0.1%. Before this move, Japan was the only country in the world to retain negative interest rates. Finance professor Patrick Boyle released a helpful video explaining the context behind the decision and how it might affect international finance and Japanβs broader economy.
ππ Amazon is ending checkout-free shopping, which had been powered by a team in India
Many of Amazonβs brick-and-mortar grocery stores are equipped with βJust Walk Outβ technology that lets you exit and pay without stopping at a checkout counter, as long as you scan a QR code on your way in. The stores used sophisticated cameras and sensors to track people and items throughout the store, but a recent exposΓ© revealed that Amazon also relied on a team of 1,000 people in India, who would watch and label video of stores to βensure accurate checkouts.β Amazon has now announced that itβs phasing out this βJust Walk Outβ technology and will replace it with shopping carts that let you scan and pay for items as you shop.
ππΈ A major AI startup ran out of money to pay its GPU bills
Stability AI, the embattled company behind the popular text-to-image model Stable Diffusion, has reportedly been paying almost $100 million a year to various cloud vendors on just $11 million a year in sales. As of last October, the company had just $4 million in the bank, and due to thwarted fundraising attempts it was unable to pay millions of dollars of cloud bills last year. (Stability has also been in the news for the resignation of its former CEO, Emad Mostaque, amidst these financial woes.)
ππ² Colorado announced the USβs first e-bike tax credit
A new bill signed into law in Colorado will give all Coloradans an instant $450 discount on purchasing electric bicycles from a participating retailer; itβs an automatic discount applied at the point of sale rather than a more cumbersome rebate. Retailers will also get $50 per qualifying e-bike sale as an incentive to sign up.
πβ³ Worth your time
Some especially insightful pieces weβve read, watched, and listened to recently.
What We Know About the XZ Utils Backdoor That Almost Infected the World (Ars Technica) β A timeline of what one expert said βmight be the best executed supply chain attackβ weβve seen on widely used open-source software; it explains the attackersβ long-con infiltration of a key repository and a teardown of how the backdoor works. (Itβs also worth reading about the βfolk heroβ software engineer who spotted the malware when he noticed that the SSH tool used a small amount more power than usual.)
OKRs Are Bullshit (David Morrison) β Observes that βOKRsβ have largely become a synonym for βplanning,β and the various interpretations of what this process looks like can have many shortcomings. Not all things can or should be measured, and not all worthwhile goals are knowable a quarter or a year ahead of time.
The Philosopher Who Critiqued Effective Altruism More Than 250 Years Ago (TIME) β Discusses how Jean-Jacques Rousseau critiqued an imaginary young man named Chrysophile who sought to amass great wealth in order to alleviate human suffering β not too different from the stated goals of the recently sentenced SBF. Rousseau warned that a paradox lay at the center of this mindset: the greed required to chase and gain wealth would change him, making it impossible to maintain his philanthropic mindset. His goal would inevitably shift toward preserving his wealth rather than distributing it.
All of Proto-Indo-European in Less Than 12 Minutes (Zzineohp) β An impressively thorough yet brief introduction to the famous ancestor of Latin, Greek, and Sanskrit, this video introduces key linguistics concepts like ablaut, lexemes, and inflection systems. By the end, you can even translate a simple PIE sentence into English.
More From FLUX
Finishing Schools, Generative AI, and how Ethics is not a solvable problem. (Adventure Capital) Ben wades into the spicy waters of AI and the culture wars but tries to raise the debate about whether any approach to Responsible AI might ever be able to address Ethics and The Good at all through the lens of Cybernetics and requisite variety.
ππ΅οΈ Lens of the week
Introducing new ways to see the world and new tools to add to your mental arsenal.
This weekβs lens: data lenses (induction, abduction, and deduction).
Todayβs lens follows from our main article and explores different ways of knowing and making inferences. Despite the vast diversity of data decision-makers may be confronted with, it's somewhat comforting that there are only three ways in which data can lead to conclusions. Thinking through which one(s) we are interested in before embarking on collection or analysis can be clarifying.
Inductive reasoning focuses on collecting data and insights from as broad a spectrum as possible β not coming to the data with a pre-formed theory/hypothesis to be proved. Inductive reasoning doesnβt create causal hypotheses from the data but relies on accumulating regularity: the more consistent a pattern has been in the past, the more likely it is to happen again. Weβre simply soaking it all in β a walk in the woods, as it were. We step on leaf after leaf, each crunching nicely. Inductively, we become gradually more convinced stepping on leaves will produce a crunch.
Abductive reasoning, to some extent, follows inductive reasoning, except it describes inference to the best explanation, arriving at a theory from the data. (In some formulations, new explanations are also formed). Data alone does not create or contain explanations, but humans constantly seek to explain data. With abduction, weβre not beginning with a single pre-formed thesis; instead, based on our exploration, we use abduction to judge the best thesis to explain the data we have. For example, if we see a flattened path in the woods and a stream of deer passes, abduction arrives at our best explanation: deer created the path! (Interestingly, though Sherlock Holmes called his methods βdeductive reasoning,β logicians think his method was closer to abductive reasoning.)
Finally, deductive reasoning allows us to take a thesis and draw conclusions from data with this in mind. In the walk-in-the-woods example, if our thesis was that all paths lead to candy houses, then deduction lets us conclude that a candy house lies ahead. To test this deductive conclusion, we can walk further. If we indeed end up at a candy-covered house, weβve now observed a regularity and inductively might believe it more strongly!
When we explore data, it can be helpful to consider which βlensβ we use. If we use the wrong lens, we might inadvertently overfit it to a forgone hypothesis. After all, if we torture the data long enough, it will confess to anything.
Β© 2024 The FLUX Collective. All rights reserved. Questions? Contact flux-collective@googlegroups.com.